Prediction: AI Gets Affordable – The Coming Drop in Transaction Costs
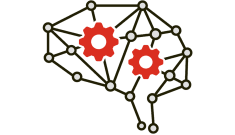
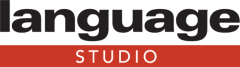
Advancing Efficiency: Algorithms and Hardware Innovations
Efficient Algorithms
Efficient algorithms are at the forefront of reducing AI transaction costs. Cutting-edge methods, such as energy-saving algorithms like L-Mul, are enabling AI systems to process data with minimal computational and energy overhead. These algorithms optimize computations like tensor multiplications and dot products, achieving significant energy savings without sacrificing accuracy. For example, some systems now report up to a 95% reduction in energy costs for key operations. See https://arxiv.org/pdf/2410.00907
Additionally, improvements in optimization techniques are allowing AI algorithms to become more robust and resource-efficient. Techniques such as quantization, pruning, and knowledge distillation are minimizing the computational load of models while maintaining performance. These optimizations reduce training times and inference costs, enabling businesses to deploy AI solutions with reduced overhead. This evolution in algorithm efficiency is critical for scaling AI across diverse industries, from healthcare diagnostics to automated customer service.
These algorithmic breakthroughs not only lower financial and environmental costs but also open doors for smaller enterprises to adopt AI solutions that were previously inaccessible due to high expenses.
Energy-Efficient Hardware
Hardware innovation is another critical factor driving down AI costs. Companies like NVIDIA and AMD are developing AI-specific chips tailored for performance and energy efficiency. For example, NVIDIA’s H100 GPU accelerates training and inference for transformer models while reducing power consumption, and AMD’s MI300 series focuses on optimizing energy usage for high-performance applications.
Energy-efficient hardware is also being designed with sustainability in mind. Emerging technologies like neuromorphic computing and application-specific integrated circuits (ASICs) are delivering unprecedented efficiency by mimicking the brain’s neural pathways or optimizing for specific AI workloads. These advancements significantly reduce power consumption while increasing computational throughput.
As competition in the hardware sector intensifies, more cost-effective solutions will emerge, democratizing access to AI capabilities. These innovations are particularly beneficial for industries like retail and logistics, where real-time AI analysis at scale is crucial but often hindered by operational costs.
Fierce Competition
The landscape of AI development is marked by fierce competition among large language model (LLM) builders and providers, driven by the proliferation of free and open-source models like Meta’s Llama. These freely available models limit the pricing power of proprietary systems, compelling developers to innovate and improve cost efficiency to maintain market relevance. Adding to the competitive pressure, academia, governments, and non-profits are contributing to the rapid advancement of AI technologies, ensuring continuous progress without significant cost barriers. This dynamic ecosystem fuels innovation while democratizing access to AI capabilities, creating a highly competitive and rapidly evolving market.
Simultaneously, LLM users are prioritizing cost minimization, focusing on models and adjustments that strike a balance between quality and affordability. Diverse use cases demand varying levels of sophistication, leading businesses to favor solutions that are “good enough” for specific tasks rather than incurring the high costs of premium performance. Cost-effective strategies, such as task-specific adjustments and leveraging open-source or on-demand platforms, are becoming integral for AI adoption. This focus on financial efficiency reflects a shift in the market, as organizations strategically tailor AI solutions to their needs without compromising budgets.
Optimization of AI Models for Cost Savings
Compact and Efficient Models
AI models are becoming smaller and more efficient while maintaining high performance. For instance, Meta’s Llama 3.3 70B model offers superior outputs compared to larger predecessors like the Llama 3.1 405B model, all at a fraction of the computational cost. These more compact models are optimized for real-world applications, such as multilingual dialogues and extended token contexts.
Smaller models are enabling businesses to achieve high-quality outcomes without the need for massive computational infrastructure. Advances in architecture design, such as sparse models and modular AI systems, allow developers to train and deploy smaller models that focus only on task-relevant parameters. This targeted approach reduces energy consumption, improves latency, and lowers the overall cost of ownership for AI systems.
Enabling Edge Computing: Expanding AI to Resource-Constrained Environments
Smaller, optimized AI models are driving the adoption of edge computing by enabling AI to operate on devices with limited hardware, such as smartphones, IoT sensors, and autonomous drones. By shifting processing tasks from centralized cloud systems to local devices, edge computing reduces latency, enhances data privacy, and cuts operational costs.
This approach is transforming industries reliant on real-time decision-making, from healthcare facilities in remote areas using AI for diagnostics to autonomous vehicles processing sensor data locally for immediate navigation. Edge computing eliminates the need for constant cloud connectivity, making AI more accessible and scalable for resource-constrained environments, while unlocking new possibilities for innovation and efficiency.
Cost-Effective Customization: RAG, Knowledge Graphs, and Open-Source Models
Rise of RAG and Knowledge Graphs: Lowering Customization Costs
The integration of Retrieval-Augmented Generation (RAG) and knowledge graphs (KG) is revolutionizing AI customization by drastically lowering costs while enhancing performance. RAG leverages external, domain-specific knowledge dynamically during AI interactions, allowing organizations to bypass expensive, full-scale fine-tuning of models. Instead of retraining a model for every unique use case, RAG enables models to pull relevant information in real time, reducing computational overhead and development time.
Knowledge graphs further amplify these benefits by structuring and interconnecting data, enabling more accurate and context-aware AI outputs. For example, in industries like legal or healthcare, KGs can organize complex datasets, allowing AI systems to deliver precise, actionable insights without needing large-scale retraining. This synergy minimizes the resources required to deploy effective, task-specific AI systems, making advanced customization accessible even to smaller organizations. By combining the strengths of RAG and KGs, businesses can achieve high-quality AI solutions tailored to their needs at a fraction of the cost traditionally associated with foundation model fine-tuning.
Adoption of Open-Source and Customizable Models
Open-source AI platforms, such as Hugging Face and AWS Bedrock, are democratizing access to powerful AI tools. By offering free or low-cost models like Meta’s Llama 3.3 70B, these platforms allow developers and organizations to fine-tune AI for specific needs without incurring hefty development costs. Similarly, EleutherAI’s GPT-NeoX provides an open-source alternative to proprietary language models, offering comparable performance while maintaining the flexibility to adapt to unique use cases.
Another example is BigScience’s BLOOM, a multilingual language model that supports over 50 languages and is freely accessible for research and commercial use. This broad linguistic support makes BLOOM a valuable resource for businesses operating in diverse global markets, enabling tailored solutions without substantial investment.
The widespread adoption of open-source frameworks is fueling a wave of innovation by lowering the barriers to entry for businesses of all sizes. Organizations can leverage these pre-trained models to accelerate their AI initiatives while allocating resources toward specific customizations. Furthermore, open-source communities foster collaboration, enabling shared solutions for common challenges and driving the evolution of AI tools at a rapid pace.
This accessibility is particularly impactful for startups and small businesses, allowing them to compete with larger enterprises in AI-driven innovation. As open-source platforms continue to expand their offerings, the affordability and versatility of AI will reach unprecedented levels.
LLM users, particularly businesses operating on tight budgets, are expected to carefully optimize expenses by balancing quality and cost. With diverse use cases demanding varying levels of AI sophistication, users are prioritizing models and customizations that are ‘good enough’ rather than excessively expensive. This shift reflects a growing emphasis on cost-effectiveness over perfection, as organizations strategically align AI solutions with specific, task-relevant needs.
Scalable and Flexible Access: AI On-Demand and Strategic Resource Allocation
AI On-Demand: Affordable Access Without Hardware Investments
On-demand AI services like AWS Bedrock, Google Cloud’s Vertex AI, and Microsoft Azure’s OpenAI Service are revolutionizing how businesses access AI capabilities. Operating on a pay-as-you-go model, these platforms provide pre-trained models and infrastructure without the need for costly hardware investments.
For small and mid-sized enterprises, this model eliminates the need for maintaining complex infrastructure, reducing both upfront and ongoing expenses. Platforms like these offer a wide variety of pre-trained models—ranging from proprietary options like Google’s PaLM and OpenAI’s GPT models to third-party offerings from Cohere, Anthropic, and others. They also include robust tools for fine-tuning and integrating AI into existing workflows, accelerating deployment timelines and reducing development complexity.
This approach is democratizing AI by enabling organizations to experiment with and scale solutions without prohibitive costs, making advanced AI technologies more accessible across diverse industries. By leveraging the strengths of platforms from multiple providers, businesses can tailor their AI strategies to best suit their unique needs and goals.
Strategic Scheduling and Resource Allocation
Businesses are increasingly leveraging renewable energy and strategic resource management to minimize AI-related costs. By scheduling computationally intensive tasks during off-peak energy periods or when renewable energy is abundant, organizations can reduce expenses and their carbon footprint.
Cloud service providers are also offering advanced tools to align AI workloads with cost-efficient energy use. For example, platforms now enable users to set preferences for running tasks based on energy pricing or availability, creating an eco-friendly and cost-effective operational model. These strategies are particularly valuable for enterprises managing large-scale AI deployments, where energy consumption represents a significant portion of operational expenses.
Implications for AI Accessibility and Adoption
The reduction in AI transaction costs is a pivotal step in democratizing access to this transformative technology. By lowering financial and operational barriers, AI is no longer a tool reserved for large enterprises. Instead, businesses of all sizes can now harness its potential to drive innovation and efficiency. Key impacts include:
- Empowering Smaller Enterprises: Cost reductions enable small and medium-sized businesses to adopt AI for tasks like automation, data analysis, and personalized services, leveling the playing field with larger competitors.
- Encouraging Innovation: Widespread access allows organizations to experiment with AI-driven solutions, fostering creativity and efficiency in areas that were previously resource-intensive.
This democratization has broader implications:
- Global Transformation: Accessible AI accelerates digital transformation across diverse organizations, driving productivity and fostering global innovation.
- Equity in Technology: Affordable AI narrows the gap between resource-rich enterprises and smaller organizations, promoting a more inclusive technological ecosystem.
As the cost of AI continues to decline, its adoption is no longer a question of “if” but “how effectively” organizations will integrate it into their operations. The democratization of AI ensures that the benefits of this technology are shared widely, reshaping industries and enabling organizations worldwide to participate in the AI-driven economy.
The Bottom Line
AI is entering a transformative era where affordability meets accessibility, revolutionizing its role across industries. Advances in algorithm efficiency, compact model designs, energy-efficient hardware, and innovative solutions like RAG and knowledge graphs are drastically reducing costs, making AI practical for organizations of all sizes. The rise of open-source frameworks and on-demand platforms further democratizes access, enabling even small businesses to leverage AI for innovation and growth without prohibitive investments.
In 2025, the convergence of these advancements will shift AI from a luxury to a universal necessity, unlocking unprecedented opportunities in efficiency, sustainability, and scalability. This new wave of affordable AI promises to empower organizations worldwide, leveling the playing field and catalyzing innovation at an unprecedented pace. The focus now shifts from “if” businesses will adopt AI to how effectively they will seize its transformative potential.