With the advances in artificial intelligence, interest has grown in the use of speech recognition and speech synthesis. So too has the number of terms and industry jargon used by professionals. We’re so enthusiastic about these AI advances that we often get carried away and forget that words can be intimidating to beginners. While there are many differences in the two technologies, there are also many similarities. For this reason we have merged our speech recognition glossary and our speech synthesis glossary into a single glossary that is perhaps best described as a speech processing glossary.
While this may not be the only voice recognition glossary and text-to-speech glossary that you will ever need, we have tried to make it as comprehensive as possible. If you have any questions about terminology or you would like to suggest some terms to be added, please contact us.
So here it is. We’ve created a glossary of terms to help you get up to speed with some of the more common terminology – and avoid looking like a novice at meetings.
Before we start…
Speech recognition and voice recognition are two distinct technologies that recognize speech/voices for different purposes, while text-to-speech (also known as speech synthesis) is the creation of synthetic speech. All technologies are closely related but different. Before we get into glossary terminology, it is important to understand the key definitions of the topics that this glossary covers:
- Speech recognition is primarily used to convert speech into text, which is useful for dictation, transcription, and other applications where text input is required. It uses natural language processing (NLP) algorithms to understand human language and respond in a way that mimics a human response.
- Voice recognition, on the other hand, is commonly used in computer, smartphone, and virtual assistant applications. It relies on artificial intelligence (AI) algorithms to recognize and decode patterns in a person’s voice, such as their accent, tone, and pitch. This technology plays an important role in enabling security features like voice biometrics, where a person’s voice is used to verify their identity.
- Text-to-Speech / Speech Synthesis is a type of technology that converts written text into spoken words. This technology allows devices, such as computers, smartphones, and tablets, to read out loud digital text in a human-like voice. TTS is often used to assist people who have difficulty reading or have visual impairments, as well as for language learning, audiobooks, and accessibility in various applications. More advanced forms of TTS are a used to synthesize human like speech, with some examples being very difficult to differentiate between a real human and a synthesized voice.
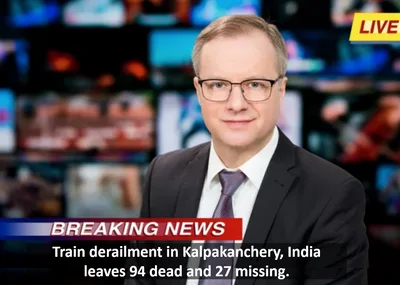
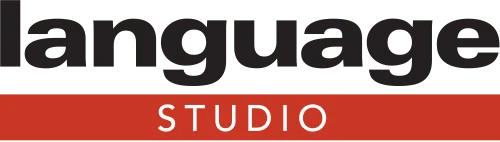
Language Studio offers a wide range of speech processing features
- Transcribe in 48 languages
- Advanced Media Processing (AMP)
- Translate Subtitles and Captions in Real-Time
- Live News and Sports Streaming
- Dictate Microsoft Office Documents
- Transcribe Video and Audio Files into Captions and Subtitles
- Multi-Language Interviews
- Real-Time transcription of Video Conferences, Calls and Webinars
- Customized Speech Recognition via Domain Adaptation
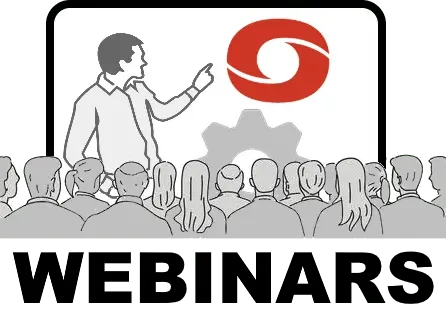
Check out our recent webinars about ASR and TTS
V
Vocabulary
Vocabulary refers to the complete set of words that a speech engine is capable of recognizing and interpreting. This set of words is used to compare against the user’s spoken utterance to determine the intended meaning of the spoken words.
The vocabulary of a speech engine is typically made up of all the words in all active grammars. A grammar is a set of rules that defines the possible word combinations and structures that can be used to express a particular meaning or intent. For example, a grammar might define the rules for ordering food at a restaurant, or for setting a reminder on a smartphone.
The size and composition of the vocabulary can have a significant impact on the accuracy and effectiveness of a speech recognition system. A larger vocabulary can allow for more accurate interpretation of a wider range of spoken utterances, but can also increase the computational complexity and processing time required to analyze the spoken words.
In addition, the vocabulary of a speech recognition system may be tailored to the specific needs and preferences of different users or applications. For example, a medical transcription system might have a specialized vocabulary that includes technical terms and jargon specific to the medical field.
Overall, the vocabulary of a speech recognition system is a critical component in enabling accurate and effective communication between humans and machines, and continues to be an area of ongoing research and development in the field of natural language processing.
Vocoder
A vocoder is a device or algorithm that analyzes speech and generates a digital representation of the speech signal. It is a type of speech coding technology that was first developed in the early 20th century and has since been used in a variety of applications, ranging from music production to military communications.
The basic principle of a vocoder is to break down a speech signal into its constituent parts, or frequency bands, and then to analyze and encode each band separately. This is accomplished using a set of filters and modulators that extract the spectral information from the speech signal and then apply it to a carrier signal, which is typically a synthetic waveform or musical instrument sound.
The output of a vocoder is a digital representation of the speech signal that can be played back through a device or system. This output can be manipulated and modified in a variety of ways to create unique and interesting effects, such as robotic or synthesized voices, or to enhance the quality and clarity of speech in noisy or crowded environments.
Vocoders have many potential applications, ranging from music production and sound design to military communications and voice encryption. They are also commonly used in speech recognition and text-to-speech systems, where they can be used to improve the accuracy and naturalness of synthesized speech.
Overall, vocoders are an important and versatile technology in the field of speech processing, with many potential applications and benefits for users and society as a whole.
Voice Activity Classification
Voice activity classification is the process of analyzing an audio signal and classifying segments of the signal as either speech or non-speech. This technology is commonly used in speech processing applications, such as speech recognition and audio transcription, to identify and extract the speech content from a larger audio stream.
The process of voice activity classification typically involves analyzing the audio signal and extracting features that are indicative of speech, such as energy levels, spectral properties, and pitch. These features are then fed into a machine learning algorithm, such as a neural network or decision tree, that has been trained on a large dataset of annotated audio samples.
The algorithm then uses these features to classify each segment of the audio signal as either speech or non-speech, based on a set of predefined criteria. For example, it may classify a segment as speech if its energy level exceeds a certain threshold and its spectral properties match those of human speech.
Voice activity classification has many potential applications, ranging from speech recognition and audio transcription to security and surveillance. It can be used to automatically identify and extract speech content from large audio streams, making it easier to analyze and understand the content of recorded conversations and other audio data.
Overall, voice activity classification is an important and widely used technique in speech processing, with many potential applications and benefits for users and society as a whole.
Voice Activity Detection
Voice Activity Detection (VAD) is a process used in speech recognition systems to identify the parts of an audio signal that contain speech. VAD is typically used to segment an audio stream into speech and non-speech regions. It is a critical component in speech recognition systems because it helps to filter out background noise and other non-speech signals that can interfere with accurate recognition.
VAD algorithms are designed to analyze the acoustic properties of an audio signal and determine whether or not speech is present. This is done by looking for specific patterns and characteristics that are common to speech signals, such as changes in frequency and energy levels. Some VAD algorithms are based on machine learning techniques and use large datasets to train the algorithm to recognize speech patterns.
VAD is used in a variety of applications, including voice-activated assistants, call center routing, and speech-to-text transcription. By accurately detecting speech, VAD can improve the accuracy and efficiency of these systems, making them more reliable and user-friendly.
Voice Biometrics
Voice Biometrics is a technology that identifies specific markers within a given piece of audio that was spoken by a human being and uses those markers to uniquely model the speaker’s voice. The technology is the voice equivalent of taking a visual fingerprint of a person and associating that unique fingerprint with the person’s identity.
Voice Biometrics technology is used for both Voice Identification and Voice Verification. In Voice Identification, the technology is used to match a given speaker’s voice against a database of known speaker voices, while in Voice Verification, the technology is used to compare a voice input against a given speaker’s voice to confirm their identity.
To perform Voice Biometrics, a system first records a speaker’s voice and extracts features such as pitch, tone, and speech patterns. These features are then compared to a previously recorded voiceprint of the same individual, which contains unique markers that identify the speaker’s voice.
Voice Biometrics can be used in a wide range of applications, from security and access control to customer service and personalized user experiences. For example, in security applications, Voice Biometrics can be used to confirm the identity of a user before granting access to a secure system, while in customer service, it can be used to personalize interactions by identifying returning customers.
As Voice Biometrics technology continues to improve, it is likely to play an increasingly important role in a wide range of applications, from security and law enforcement to personalized customer experiences and beyond. However, it is important to note that Voice Biometrics technology is not foolproof and can be subject to false positives and false negatives, which can have serious implications in some applications. Therefore, careful consideration must be given to the use and implementation of Voice Biometrics technology in different contexts.
Voice Chat Moderation API
A Voice Chat Moderation API, also known as real-time voice chat moderation API, is a technology that allows for the connection between an ongoing voice chat (such as video game voice chat or voice chat in the metaverse) and processes the spoken language in real-time in order to identify instances of hate speech, harassment, or other inappropriate behavior.
The API uses machine learning algorithms to analyze the audio stream in real-time and flag any instances of inappropriate behavior. This can include detecting abusive language, hate speech, threats, or other types of harmful behavior. The system can then take appropriate action, such as issuing a warning or alerting a moderator to step in and address the situation.
One of the key benefits of a Voice Chat Moderation API is that it allows for real-time monitoring and intervention, which can help to prevent harmful behavior from escalating. It can also help to create a safer and more welcoming environment for all participants in the voice chat.
The use of Voice Chat Moderation APIs is becoming increasingly important as more people engage in online voice chats and virtual environments, where it can be difficult to monitor behavior and ensure that everyone is following the rules of conduct. By using machine learning algorithms and real-time monitoring, Voice Chat Moderation APIs can help to create a safer and more inclusive online environment for everyone.
Voice Cloning
Voice cloning is the process of creating a synthetic voice that is designed to resemble a specific human voice. This technology uses advanced algorithms and artificial intelligence (AI) techniques to analyze the unique characteristics of a person’s voice, such as tone, pitch, and pronunciation, and generate a digital model that can mimic their speech patterns.
Voice cloning can be used for a variety of purposes, such as creating realistic voiceovers for videos, enhancing the accuracy of speech recognition systems, and even allowing people with speech impairments to communicate more easily using a synthesized voice that sounds like their own.
The process of voice cloning typically involves recording a large amount of audio data from the target voice, which is then analyzed and processed by the AI model to create a digital representation of the voice. This representation can be fine-tuned and customized to suit different applications and use cases, such as changing the tone or style of the synthesized voice to better match a specific context or audience.
While voice cloning technology has many potential benefits, there are also concerns about its potential misuse, such as creating synthetic voices for fraudulent or malicious purposes, such as impersonating others or spreading disinformation. As such, there is a growing need for ethical guidelines and regulations to govern the development and use of voice cloning technology.
Voice Conversion
Voice conversion is the process of modifying the acoustic characteristics of a voice to make it sound like another speaker. This technology uses advanced signal processing and machine learning techniques to analyze the acoustic properties of a target voice and then modify the source voice to match those properties, resulting in a synthesized voice that sounds like the target speaker.
The process of voice conversion typically involves recording a sample of the source speaker’s voice and a sample of the target speaker’s voice, and then extracting and analyzing the acoustic features of both samples, such as pitch, spectral envelope, and phonetic content. These features are then used to create a mapping between the source and target voices, which can be used to transform the source voice to sound like the target speaker.
Voice conversion has many potential applications, ranging from entertainment and artistic expression to speech therapy and language learning. It can be used to create realistic and expressive synthesized voices for film and video game characters, or to help people with speech disorders or disabilities to communicate more effectively.
Voice conversion can also be used for security and forensics purposes, such as identifying anonymous or disguised speakers in recorded conversations. However, there are also concerns about the potential misuse of this technology, such as the creation of fake audio recordings for impersonation or manipulation purposes.
Overall, voice conversion is an exciting and rapidly evolving area of speech technology, with many potential applications and benefits for users and society as a whole. It represents a significant advancement in our ability to manipulate and modify the sound of human speech, and has the potential to transform many aspects of our lives and our interactions with technology.
Voice-Enabled Assessment
Voice-based assessment is a method that uses speech recognition technology to listen, analyze and evaluate a child’s reading skills as they read aloud.
The significance of this technology lies in its ability to provide accurate data on a child’s pronunciation, reading speed, and fluency, both in the classroom and remotely. Additionally, it can be used to screen for learning difficulties such as dyslexia.
By using speech recognition technology to assess a child’s reading skills, educators can gather data to better understand a child’s progress and determine the level of support required. This technology can improve educational outcomes for children, as well as provide valuable insights into how to better support their learning journey.
Voice First
Voice First refers to interfaces or applications where the primary interface between the user and the system is based on voice. The term is similar in concept to mobile-first, which refers to designing applications for mobile devices before desktop or other platforms.
However, being Voice First does not necessarily mean being “Voice Only”. A Voice-First interface can have an additional, adjunct interface (usually a visual one) that supplements the user experience. For example, a user can ask a Voice-First system if the nearest post office is open, receive the answer verbally, and then be provided with additional details about the post office location on a visual interface, such as a mobile app or desktop browser.
The goal of a Voice-First approach is to create a user experience that is primarily based on voice interactions, rather than relying on traditional interfaces such as text or touch. This approach can be particularly useful in situations where users have their hands occupied, such as while driving or cooking, or for individuals with limited mobility or vision.
Voice-First interfaces can be implemented using various technologies, such as voice assistants, chatbots, or speech recognition systems. These systems can be integrated into a wide range of devices, including smartphones, smart speakers, and other smart home devices.
Overall, the Voice-First approach has the potential to provide a more natural and intuitive user experience, while also enabling greater accessibility and convenience for users. However, designing effective Voice-First interfaces requires careful consideration of the unique challenges and opportunities posed by voice interactions, as well as a deep understanding of user needs and preferences.
Voice Font
A voice font is a digital representation of a particular human voice that can be used in text-to-speech systems. It is a type of speech synthesis technology that enables computer-generated voices to sound more natural and expressive, as if they were speaking with a specific human voice.
Voice fonts are created by recording a large amount of speech data from a human speaker and then using machine learning algorithms to analyze and model the unique vocal characteristics of that voice. This process involves capturing different aspects of speech, such as pitch, intonation, and cadence, and mapping them to corresponding digital parameters that can be used to generate speech in real-time.
Once a voice font has been created, it can be integrated into a text-to-speech system, where it can be used to generate speech that sounds like the original human speaker. This technology has many potential applications, such as providing more personalized and engaging user experiences for virtual assistants, automated customer service systems, and other digital interfaces.
Voice fonts can also be used to preserve and replicate the voices of individuals who may be at risk of losing their ability to speak due to medical conditions or other reasons. By creating a digital copy of their voice, they can continue to communicate with others in a way that sounds like their own natural voice, even if they are unable to speak.
Overall, voice fonts are an exciting and rapidly evolving area of speech technology, with many potential applications and benefits for users and society as a whole.
Voice Identification
Voice Identification, also known as speaker recognition, is the capability of identifying a specific speaker’s identity among a list of possible speaker identities based on the characteristics of the speaker’s voice input. Voice ID systems are trained by being provided with samples of speaker voices and then using machine learning algorithms to recognize unique characteristics of each speaker’s voice.
Voice Identification technology has a wide range of applications, from law enforcement and security to customer service and personalized user experiences. In law enforcement, for example, Voice Identification can be used to match a suspect’s voice to a recording of a crime, while in customer service, it can be used to personalize interactions by identifying returning customers.
To perform voice identification, a system first records a speaker’s voice and extracts features such as pitch, tone, and speech patterns. These features are then compared to a database of known speaker voices to determine the likelihood of a match. Voice Identification systems can be trained on a large dataset of speaker voices to improve accuracy and reduce false positives.
One of the key challenges in Voice Identification is dealing with variability in the speaker’s voice due to factors such as age, gender, accent, and emotional state. To address this, Voice Identification systems can be trained to recognize patterns of variation and adapt to individual speakers over time.
As Voice Identification technology continues to improve, it is likely to play an increasingly important role in a wide range of applications, from security and law enforcement to personalized customer experiences and beyond.
Voice Morphing
Voice morphing refers to the process of transforming one voice into another by altering its acoustic characteristics. This technology uses advanced signal processing algorithms to modify the fundamental frequency, spectral envelope, and other acoustic features of a recorded voice, in order to make it sound like a different person.
Voice morphing has a variety of applications, ranging from entertainment and creative expression to practical uses in security and law enforcement. In entertainment, voice morphing can be used to create realistic voiceovers for animated characters, or to produce unique and creative sound effects for music and film. In security and law enforcement, voice morphing can be used to disguise the identity of a speaker, or to enhance the accuracy of voice recognition systems by improving the quality and diversity of training data.
The process of voice morphing typically involves recording a large amount of speech data from the target voice, as well as the source voice that will be used to transform it. This data is then analyzed and processed using specialized algorithms that can identify and manipulate the key acoustic features of each voice. By selectively modifying these features, it is possible to create a digital model that can transform the source voice into a close approximation of the target voice.
While voice morphing can be a powerful and useful technology, it can also be misused for fraudulent or malicious purposes. For example, it can be used to impersonate someone else in order to commit identity theft, or to create fake audio recordings that spread false information or defame individuals. As such, there is a growing need for ethical guidelines and regulations to govern the use and development of voice morphing technology, in order to protect individuals and society from potential harm.
Voice Persona
Voice persona refers to the unique character and personality conveyed by a synthetic voice in a text-to-speech system. It is the collection of vocal characteristics and nuances that give a voice its distinctive style, tone, and emotional expressiveness, and that help to create a more engaging and personalized experience for the listener.
Voice persona can be created through a variety of techniques, such as adjusting the pitch, intonation, and rhythm of the synthesized voice, as well as adding specific inflections, pauses, and other vocal cues that help to convey different emotions and attitudes. By carefully crafting the voice persona of a synthetic voice, it is possible to create a more compelling and effective communication experience, whether for marketing, education, or other purposes.
Voice persona is an important consideration in the design and development of text-to-speech systems, as it can have a significant impact on user engagement and satisfaction. A voice with a strong and distinctive persona can help to create a more memorable and enjoyable experience for the listener, while a voice that sounds robotic or impersonal may have the opposite effect.
Overall, voice persona is an important aspect of speech technology, with many potential applications in areas such as marketing, education, and entertainment. By creating synthetic voices that have a strong and engaging persona, it is possible to enhance the effectiveness and appeal of text-to-speech systems, and to provide more personalized and satisfying user experiences.
Voice Quality
Voice quality refers to the acoustic characteristics of a voice, including its pitch, tone, timbre, and other sound qualities. These features determine the unique sound of a person’s voice and play an important role in conveying meaning and emotion in spoken communication.
Pitch refers to the frequency of a person’s voice, or how high or low it sounds. Tone refers to the emotional or expressive quality of a voice, such as whether it sounds happy, sad, angry, or neutral. Timbre refers to the unique sound of a voice, which is determined by the combination of its fundamental frequency and the harmonics that make up the sound wave.
Voice quality is an important consideration in many areas of speech technology, such as text-to-speech systems, voice recognition systems, and speech therapy. For example, in a text-to-speech system, the voice quality of the synthesized voice can have a significant impact on how engaging and effective the system is for users. In voice recognition systems, voice quality can affect the accuracy of the system in identifying and verifying the speaker’s identity.
Speech therapy also places a significant emphasis on voice quality, as it can be used to diagnose and treat various voice disorders, such as vocal nodules, vocal cord paralysis, and spasmodic dysphonia. By analyzing the acoustic characteristics of a person’s voice and providing targeted therapy to improve their voice quality, it is possible to help them overcome these disorders and improve their overall communication ability.
Overall, voice quality is a crucial aspect of spoken communication, and is an important consideration in many areas of speech technology and therapy. By understanding and manipulating the acoustic features of a voice, it is possible to create more engaging and effective communication experiences for users, as well as to diagnose and treat voice disorders more effectively.
Voice Recognition
Voice recognition, also known as speaker recognition, is the process of identifying a speaker based on their unique voice characteristics. This technology uses advanced algorithms and machine learning techniques to analyze and compare acoustic features of a person’s voice, such as pitch, intonation, and speech patterns, to identify and verify their identity.
Voice recognition can be used for a variety of purposes, such as access control, security, and forensics. In access control, voice recognition can be used to authenticate users and provide secure access to sensitive data or systems. In security, voice recognition can be used to identify and track suspects based on their voice, or to monitor and analyze large amounts of audio data for suspicious activity. In forensics, voice recognition can be used to analyze recorded conversations and identify the speakers involved in a crime.
The process of voice recognition typically involves creating a voiceprint, which is a digital representation of a person’s unique voice characteristics. This voiceprint is generated by recording a sample of the person’s voice, which is then analyzed and processed by the voice recognition system to extract and store the relevant acoustic features. When a person speaks again, the system can compare the new voiceprint to the stored voiceprint and determine if they match, allowing for accurate identification and verification.
While voice recognition technology has many potential benefits, there are also concerns about its potential misuse, such as the use of voice recordings for impersonation or the violation of privacy rights. As such, there is a growing need for ethical guidelines and regulations to govern the use and development of voice recognition technology, in order to protect individuals and society from potential harm.
Voice Recognition Software
Voice Synthesis
Voice synthesis, also known as speech synthesis or text-to-speech (TTS), is the process of creating artificial speech that sounds like a particular human voice. This technology uses advanced algorithms and machine learning techniques to convert written text into spoken language, and then generate a digital representation of that speech that can be played back through a device or system.
Voice synthesis can be used for a variety of purposes, such as providing accessibility for people with visual impairments, creating more engaging and interactive experiences for users of virtual assistants or other digital interfaces, and enhancing the quality and diversity of training data for speech recognition systems.
The process of voice synthesis typically involves selecting a voice font or synthetic voice that closely matches the desired characteristics of the target voice. The voice font is then used to generate the speech in real-time, using specialized algorithms to modulate the pitch, volume, and other acoustic features of the synthesized speech to make it sound more natural and expressive.
Voice synthesis technology has come a long way in recent years, and many TTS systems are now capable of producing speech that is virtually indistinguishable from human speech in terms of clarity, tone, and emotional expressiveness. This has opened up many new possibilities for enhancing the accessibility and user experience of digital interfaces, and has also created new opportunities for creative expression in areas such as music, film, and gaming.
Overall, voice synthesis is an exciting and rapidly evolving area of speech technology, with many potential applications and benefits for users and society as a whole.
Voice Transformation
Voice transformation is the process of modifying a voice to change its tone, pitch, or other characteristics using advanced signal processing techniques. This technology enables users to manipulate and customize the sound of a voice for a variety of creative and practical purposes.
Voice transformation can be used for a variety of applications, such as creating unique and interesting sound effects for music and film, altering the pitch and tone of a voice for anonymity or privacy reasons, or enhancing the emotional expressiveness of a voice for marketing or advertising purposes.
The process of voice transformation typically involves recording the original voice and then using specialized algorithms to modify its acoustic features. This can include changing the pitch, speed, or volume of the voice, as well as adding specific effects such as reverb, echo, or distortion. By selectively manipulating these features, it is possible to create a customized and unique sound that can convey a wide range of emotions and attitudes.
Voice transformation technology has advanced significantly in recent years, and many advanced systems are now capable of producing highly realistic and expressive vocal effects. This has opened up many new possibilities for creative expression in areas such as music production, sound design, and digital media, as well as for practical applications in areas such as speech therapy and voice rehabilitation.
Overall, voice transformation is an exciting and rapidly evolving area of speech technology, with many potential applications and benefits for users and society as a whole.
Voice User Interface (VUI)
A Voice User Interface (VUI) is a type of user interface that enables users to interact with electronic devices through spoken commands or responses. VUIs are the voice equivalent of Graphical User Interfaces (GUIs), which use visual elements such as buttons, icons, and menus to enable users to interact with devices.
VUIs use technologies such as speech recognition and text-to-speech to understand spoken commands and provide audible responses. They are becoming increasingly common in a wide range of electronic devices, including smartphones, smart speakers, and home automation systems.
One of the main advantages of VUIs is that they allow users to interact with devices hands-free, which can be particularly useful in situations where the user’s hands are occupied or when it is difficult to use a GUI. VUIs are also valuable for individuals with disabilities or impairments that make it difficult to use a traditional GUI.
VUIs can be found in a wide range of applications, from voice-activated personal assistants such as Apple Siri and Google Assistant to voice-activated home automation systems such as Amazon Alexa and Google Home. They are also increasingly being used in industries such as healthcare, where they can help doctors and nurses access patient information and perform other tasks more efficiently.
As VUI technology continues to advance, it is likely that we will see even more widespread adoption of voice-enabled devices and applications in the future, making it easier than ever for people to interact with technology using natural language.
Voice Verification
Voice Verification is a technology that allows for the confirmation of an individual’s identity based on their voice input. Unlike Voice Identification, which attempts to match a speaker’s voice against a database of known voices, Voice Verification compares a voice input against a given speaker’s voice and provides a match score indicating the likelihood of a match.
Voice Verification is often used in situations where there is a need for secure authentication, such as in banking or financial transactions, access control to restricted areas or information, and other identity verification applications. In a Voice Verification process, the user makes an identity claim and is then challenged to verify their identity by speaking a pre-determined phrase or set of words.
The technology works by analyzing the unique characteristics of the speaker’s voice, such as pitch, tone, and speech patterns, and comparing them to a previously recorded voiceprint of the same individual. A match score is then calculated based on the degree of similarity between the two voiceprints. If the match score is above a predetermined threshold, the user’s identity claim is verified, and access or authorization is granted.
Voice Verification technology has become increasingly sophisticated in recent years, thanks to advances in machine learning and artificial intelligence. These technologies allow for more accurate and reliable voice verification, even in challenging environments with high levels of background noise or other interfering factors.
Overall, Voice Verification is a powerful tool for secure identity verification and access control, and its use is likely to continue to grow as more organizations seek to enhance their security protocols and protect against identity fraud and other forms of cybercrime.
Voicebot
Voicebot is a type of conversational AI technology that enables users to interact with software or services using natural language through voice commands. The technology uses speech recognition and natural language processing (NLP) to understand and interpret user speech and provide a relevant response.
Voicebots can be designed for a variety of use cases such as customer service, personal assistant, healthcare, education, and more. They can be integrated into various devices such as smartphones, smart speakers, cars, and home appliances. Voicebot technology is rapidly growing in popularity due to its convenience and accessibility, particularly for users who prefer hands-free or visual-free interactions.
To create a voicebot, developers must first define the user’s intention and the corresponding set of actions to fulfill that intention. They also need to define the voice user interface (VUI) or the way the voicebot communicates with users. The VUI should be designed to provide a natural and intuitive conversation flow that makes it easy for users to interact with the voicebot.
Voicebots can be enhanced with additional features such as speech synthesis, emotional analysis, and sentiment analysis, to improve the user experience. Speech synthesis, also known as text-to-speech (TTS), allows voicebots to respond with human-like speech. Emotional analysis and sentiment analysis can help the voicebot understand the user’s emotions and provide appropriate responses.
Overall, voicebot technology is a promising field with vast potential for enhancing the way humans interact with technology and improving the efficiency of various tasks and services.
Voiceprint
Voiceprint, also known as speaker recognition, is a biometric technology that uses mathematical algorithms to identify and verify the unique characteristics of an individual’s voice. Voiceprints are created by analyzing a person’s voice, including factors such as pitch, tone, rhythm, and accent. These features are then converted into a digital representation that can be compared to a stored voiceprint for authentication purposes.
Voiceprints are used in various applications, including security systems, customer service, and personal devices. By analyzing the speaker’s voice, voiceprint technology can verify the speaker’s identity and provide secure access to sensitive information, such as bank accounts or personal data. It can also be used for fraud detection, identifying fraudulent activities by analyzing the voiceprints of suspects.
One advantage of voiceprint technology is that it is non-intrusive and does not require physical contact or interaction with the user. Additionally, voiceprint authentication can be done in real-time, making it an efficient and convenient method for authentication. However, it is important to note that voiceprint technology is not foolproof and can be susceptible to errors or spoofing attempts. As a result, voiceprint technology is often used in conjunction with other forms of authentication, such as passwords or biometric data.
W
Wake Word
A wake word, also known as a trigger word or hot word, is a specific word or phrase that serves as the activation signal for an always-listening device. Popular examples of wake words include “OK Google” or “Hey Alexa”. The purpose of the wake word is to differentiate intentional voice commands addressed to the device from regular human-to-human conversation, preventing it from recording or processing unintentional speech.
Once the device detects the wake word, it starts recording the user’s speech and begins processing the voice command that follows. Wake words are an essential component of speech recognition technology, enabling users to interact with their devices hands-free and perform various tasks such as controlling smart home devices, making phone calls, or playing music.
The effectiveness of a wake word is crucial to the overall user experience of the device, as false activations or missed activations can lead to frustration and a loss of trust. Wake words are typically customized to each device and can be changed or personalized by users to better suit their needs. They are also an important consideration for developers and manufacturers of voice-enabled devices, as choosing the right wake word can greatly impact the success and adoption of their product.
Watson
Watson, also known as IIBM Watson, is a sophisticated question-answering computer system developed by IBM’s DeepQA project. Watson is designed to analyze and interpret complex data using natural language processing, machine learning, and other advanced technologies. The system is named after Thomas J. Watson, the founder of IBM.
Watson gained widespread recognition in 2011 when it competed on the quiz show Jeopardy! against two of the show’s all-time champions, Ken Jennings and Brad Rutter. Watson was able to defeat both champions, showcasing its ability to process and understand natural language questions and respond with accurate answers in a matter of seconds.
Beyond its initial success on Jeopardy!, IBM Watson has been deployed in a wide range of industries and applications, including healthcare, finance, customer service, and more. The system has been used to help doctors diagnose diseases, assist financial analysts in making investment decisions, and even create personalized recipes based on user preferences and dietary restrictions.
IBM Watson has become a leading example of the potential of artificial intelligence and natural language processing in solving complex problems and delivering value to businesses and consumers alike. The system continues to be refined and improved upon by IBM and other developers, paving the way for new and innovative applications of AI and machine learning in the years to come.
Weak AI
Weak AI, also known as narrow AI, is a type of artificial intelligence that is designed to perform a specific task or set of tasks within a limited domain. Weak AI is contrasted with strong AI, which is a hypothetical form of AI that would be capable of performing any intellectual task that a human can.
Examples of weak AI include speech recognition systems, image recognition systems, and recommendation engines. These systems are designed to perform a specific task, such as transcribing speech, recognizing faces in images, or suggesting products based on user behavior.
While weak AI is limited in its capabilities, it has proven to be highly effective in solving specific problems and performing specialized tasks. Many of the most successful AI applications today, such as virtual assistants and autonomous vehicles, are examples of weak AI systems that are highly optimized for specific tasks.
Overall, weak AI represents a major breakthrough in the field of artificial intelligence, enabling machines to perform tasks that were once thought to be the exclusive domain of human intelligence. While there is still much to be learned and discovered in the field of AI, weak AI represents an important step forward in the development of intelligent machines.
Weight
Weight in machine learning refers to the importance or significance of a feature in a model. Each feature in a model is assigned a weight, which reflects how much it contributes to the output of the model.
The weight of a feature is determined during the training phase of a machine learning algorithm, where the algorithm learns the optimal values of the weights that minimize the error between the predicted output and the actual output. The weights are adjusted during training to find the optimal combination of features that best fits the training data.
If the weight of a feature is zero, it means that the feature has no contribution to the model and can be removed without affecting the performance of the model. Features with higher weights are considered more important and have a greater impact on the output of the model.
In some machine learning algorithms, such as linear regression and logistic regression, the weights are used to make predictions by multiplying the values of the input features by their respective weights and summing the results. The resulting sum is then passed through a function to produce the output of the model.
Overall, weights are an important concept in machine learning, as they determine the contribution of each feature to the model and can be used to make predictions and classify data.
WER
See Word Error Rate.
Word Accuracy (WAcc)
Word accuracy, also known as WAcc, is a metric used to evaluate the performance of speech recognition systems. It measures the percentage of correctly recognized words in a given speech input.
The WAcc metric is calculated as the percentage of correctly recognized words out of the total number of words in the input. For example, if the speech recognizer correctly recognizes 90 out of 100 words in the input, the WAcc would be 90%.
However, it should be noted that WAcc can sometimes be a misleading metric, especially when evaluating speech recognition systems on short or noisy inputs. In such cases, the WAcc can be artificially inflated or deflated, leading to inaccurate assessments of system performance.
As a result, the Word Error Rate (WER) is a more commonly used metric for evaluating speech recognition systems. WER measures the percentage of incorrect words in the output compared to the reference text. WER takes into account not only the correctly recognized words but also the incorrectly recognized words, making it a more robust and reliable metric for evaluating speech recognition systems.
Overall, while WAcc can be a useful metric for evaluating speech recognition systems in certain contexts, the WER metric is generally preferred for its greater accuracy and reliability.
Word Confusion Network (WCN)
A word confusion network (WCN) is a representation used in speech recognition to model the possible confusion between similar-sounding words. It is a graph-based data structure that captures the possible paths that a speech recognition system can take when it encounters ambiguous or uncertain speech input.
The basic idea behind a WCN is to model the probability distribution of different word sequences that could have produced the observed speech input, based on the acoustic and language models used by the speech recognition system. This distribution can be represented as a network of nodes and edges, where each node corresponds to a possible word hypothesis, and each edge represents a possible transition between two word hypotheses.
WCNs are useful in speech recognition systems because they can help to improve the accuracy and robustness of the system in the presence of noise, accents, or other factors that can cause errors or ambiguity in the speech input. By modeling the possible confusion between similar-sounding words, WCNs can provide a more nuanced and flexible representation of the possible word sequences that could have produced the observed speech input, and can help to disambiguate the most likely word sequence based on contextual and linguistic information.
WCNs are commonly used in speech recognition systems that operate in noisy or challenging environments, such as in-car systems, call centers, and voice assistants. They are also an active area of research in speech technology, with many potential applications and benefits for users and society as a whole.
Word Embeddings
Word embeddings are a type of natural language processing technique used to represent words as dense vectors in a high-dimensional space. These vectors capture the meaning of a word based on its context, and are often used in various NLP applications such as sentiment analysis, text classification, and machine translation.
The technique works by analyzing large amounts of text data and identifying patterns in the way words are used together. This information is then used to map each word to a unique vector in a high-dimensional space, where similar words are represented by vectors that are close to each other. This allows for efficient computation and enables machine learning algorithms to learn the relationships between words and their context.
Word embeddings have many advantages over traditional approaches to representing words, such as one-hot encoding, which can be very high-dimensional and sparse. Word embeddings are also able to capture the semantics of words, allowing them to capture relationships such as synonyms and antonyms.
Word embeddings can be trained using a variety of techniques, including neural networks like word2vec and GloVe. These models take in large amounts of text data and use unsupervised learning to learn word embeddings based on the co-occurrence of words in context. Once trained, these models can be used to generate word embeddings for new words or to improve the performance of other NLP models.
Word Error Rate (WER)
Word Error Rate (WER) is a metric used to measure the accuracy of an automatic speech recognition (ASR) system or a machine translation (MT) system. WER is calculated by comparing the words generated by the ASR or MT system with the correct transcription or translation of the input speech or text. WER is expressed as a percentage of the total number of words in the transcription or translation.
The formula for calculating WER is as follows:
WER = (S + I + D) / N
where S is the number of substitutions (words in the generated output that differ from the correct words), I is the number of insertions (words in the generated output that are not present in the correct words), D is the number of deletions (words in the correct words that are not present in the generated output), and N is the total number of words in the correct transcription or translation.
For example, if the correct transcription contains 100 words and the ASR system generates an output that contains 10 substitutions, 5 insertions, and 3 deletions, the WER would be:
WER = (10 + 5 + 3) / 100 = 0.18 or 18%
Lower WER scores indicate higher accuracy of the ASR or MT system.
Words Correct Per Minute (WCPM)
Words Correct Per Minute (WCPM) is a commonly used measure of oral reading fluency that is obtained by dividing the total number of words read correctly by the amount of time taken to read the passage. WCPM is frequently used as a measure of students’ reading proficiency, particularly in the context of assessing progress over time.
WCPM is obtained using a variety of methods, including manual counting by teachers or assistants and more automated systems that use speech recognition technology to assess students’ reading. The latter method can be particularly useful in classroom settings, as it allows teachers to quickly and easily gather data on student progress without having to dedicate additional time and resources to manual assessment.
In addition to its usefulness in assessing students’ reading proficiency, WCPM can also be used to identify areas in which students may be struggling and to guide the development of targeted interventions and support strategies. By providing a quick and objective measure of reading ability, WCPM can help teachers to better support their students and promote positive learning outcomes.
X
XML
XML stands for eXtensible markup language, a language that describes the structure of other languages. XML is useful because it allows you to exchange information between programs and systems that have different formats, which is important when data needs to be shared between different applications or systems.”
XML is the underlying data structure used in many programming languages and applications to store and exchange data. XML supports the definition of new tags, so it can be customized to the characteristics of various hardware and software platforms.
Y
Z
Zerospeech
Zerospeech is a research field in speech processing that aims to understand the underlying structure of speech without relying on phonetic or linguistic knowledge. It is an approach to speech analysis that focuses on the acoustic properties of speech signals, rather than on the phonetic or semantic content of the speech.
Zerospeech researchers use advanced machine learning and signal processing techniques to analyze large datasets of speech recordings and extract patterns and structures that are not dependent on any specific language or linguistic system. By studying these patterns, they aim to uncover the fundamental building blocks of speech and better understand how humans process and produce speech.
One of the main goals of zerospeech research is to develop more robust and accurate speech recognition systems that can adapt to different languages, dialects, and speaking styles. By identifying universal features of speech that are not dependent on any specific language or dialect, zerospeech researchers hope to develop more generalizable models of speech that can be used across a wide range of applications and contexts.
Zerospeech research also has potential applications in areas such as speech therapy, language learning, and human-robot interaction, where a deeper understanding of the underlying structure of speech can lead to more effective and personalized interventions.
Overall, zerospeech is an exciting and rapidly evolving area of speech technology, with many potential applications and benefits for users and society as a whole. It represents a shift in focus from the linguistic and semantic content of speech to its acoustic properties, and has the potential to transform our understanding of how humans process and communicate through speech.
Zero Crossing Rate
The zero crossing rate is a measure of the frequency at which an audio signal changes from positive to negative or vice versa. It is defined as the number of times that the signal crosses the horizontal axis, or zero level, per unit of time.
The zero crossing rate is an important acoustic feature of speech and music signals, as it can provide valuable information about the pitch and timbre of the signal. For example, signals with a high zero crossing rate, such as fricatives or plosives in speech, tend to have a high-frequency content and a more turbulent sound quality. In contrast, signals with a low zero crossing rate, such as sustained notes in music, tend to have a smoother and more harmonic sound quality.
The zero crossing rate can be calculated using a variety of signal processing techniques, such as Fourier analysis, wavelet transforms, or time-domain analysis. It is often used in applications such as speech recognition, music analysis, and digital signal processing, where it can help to identify and extract key features of the signal.
Overall, the zero crossing rate is an important and widely used measure of the acoustic properties of speech and music signals, with many potential applications and benefits for users and society as a whole.
Zero Resource Speech Processing
Zero resource speech processing is a field of research that explores how to perform speech processing tasks without any training data. This approach to speech processing is motivated by the fact that in many languages and dialects, there is a lack of annotated speech data, which makes it difficult to develop accurate speech recognition and synthesis systems.
The basic principle of zero resource speech processing is to use unsupervised learning algorithms to extract patterns and structures from raw speech signals, without relying on any linguistic or phonetic knowledge. This can involve techniques such as clustering, dimensionality reduction, and deep learning, which can be used to identify and extract relevant features from the speech signal.
The goal of zero resource speech processing is to develop more robust and scalable speech processing systems that can adapt to different languages and dialects, and that can operate in low-resource environments. This has important implications for areas such as speech recognition, speech synthesis, and language learning, where the ability to operate with limited data resources can be critical for success.
Zero resource speech processing is still a relatively new and rapidly evolving field, with many exciting research directions and potential applications. While it presents significant technical challenges, it also offers the promise of more flexible, adaptive, and accessible speech processing systems, which could have a transformative impact on how we communicate and interact with technology.
Zero-Shot Learning
Zero-shot learning is a type of machine learning where a model can recognize and generalize to new classes or tasks without being explicitly trained on them. Instead, the model learns from related tasks or classes during training and is able to use that knowledge to make predictions on new, unseen data.
In zero-shot learning, the model is typically trained on a set of known classes or tasks and is given a set of attributes or semantic descriptions for each class or task. These attributes or descriptions can then be used to infer relationships between the different classes or tasks, and the model can use this information to recognize and classify new examples that belong to unseen classes or tasks.
This approach is particularly useful in cases where obtaining labeled training data for new classes or tasks is difficult or expensive. It also allows for more flexible and adaptive machine learning models that can continue to improve and generalize to new scenarios over time.
Zero-Shot Speaker Recognition
Zero-Shot Speaker Recognition is a type of speaker recognition approach that allows a model to recognize new speakers without the need for explicit training data. This is achieved by training the model on a large dataset that includes a diverse set of speakers, and using this training to develop a model that can generalize to new speakers. The key advantage of zero-shot speaker recognition is that it allows for rapid adaptation to new speakers without the need for retraining the model. This approach has potential applications in a wide range of areas, including security, speech recognition, and human-computer interaction. However, it is important to note that zero-shot speaker recognition is still an active area of research, and further studies are needed to determine the best approaches for developing robust and accurate models.
Zipf’s Law
Zipf’s law is a statistical law that states that the frequency of a word in a natural language is inversely proportional to its rank in the frequency table. In other words, the most common words in a language occur much more frequently than less common words, and this relationship follows a predictable mathematical pattern.
Zipf’s law was first observed in the early 20th century by linguist George Zipf, who noted that the frequency of words in written texts follows a power law distribution. This means that the frequency of a word is proportional to its rank raised to a negative power, with the exponent typically falling between 1 and 2.
Zipf’s law has been observed in many natural languages, including English, Spanish, and Japanese, and is thought to reflect the underlying structure of human language and communication. It has important implications for many areas of linguistics and natural language processing, such as language modeling, text classification, and machine translation.
For example, Zipf’s law can be used to develop more accurate language models by weighting the most common words more heavily than less common words. It can also be used to develop more effective text classification algorithms by identifying key words and phrases that are most indicative of a particular category or topic.
Overall, Zipf’s law is an important concept in linguistics and natural language processing, and provides valuable insights into the structure and dynamics of human language.
Related Links
Pages
- Introduction to Machine Translation at Omniscien
- Hybrid Neural and Statistical Machine Translation
- Custom Machine Translation Engines
- Powerful Tools for Data Creation, Preparation, and Analysis
- Clean Data Machine Translation
- Industry Domains
- Ways to Translate
- Supported Languages
- Supported Document Formats
- Deployment Models
- Data Security & Privacy
- Secure by Design
- Localization Glossary - Terms that you should know
Products
- Language Studio
Enterprise-class private and secure machine translation. - Media Studio
Media and Subtitle Translation, Project Management and Data Processing - Workflow Studio
Enterprise-class private and secure machine translation.
FAQ
- FAQ Home Page
- Primer; Different Types of Machine Translation
- What is Custom Machine Translation?
- What is Generic Machine Translation?
- What is Do-It-Yourself (DIY) Machine Translation?
- What is Hybrid Machine Translation?
- What is Neural Machine Translation?
- What is Statistical Machine Translation?
- What is Rules-Based Machine Translation?
- What is Syntax-Based Machine Translation?
- What is the difference between “Clean Data MT” and “Dirty Data MT”?