Omniscien » Language Studio » Features » ASR »
Accuracy through Innovation and Customization
Autonomous Speech Recognition (ASR) is a new approach to automated speech recognition development that is delivering unprecedented accuracy and inclusion.
ASR uses the latest techniques in artificial intelligence and machine learning, including the introduction of self-supervised learning models, allowing for much larger datasets from a broader set of voices. Previously unutilized voice recordings from thousands of speakers can now be utilized, even those with different accents, age, or genders, reducing bias, and improving inclusion while delivering unprecedented accuracy, control and customization.
Combined all of the above with the ability to rapidly customized the ASR engine using your own custom language models and glossaries, accuracy can be further improved via fine-tuning to your own specific and unique domain.
What all this translates to is greater accuracy, faster language development and improvement, and greater control and customization. Just as importantly, it also come with greater inclusion of accents, cultures and genders – many of which bias and lack of data had previously excluded. ASRs application and purpose can be further expanded to reach all the corners of the world.
Learn more about what makes our ASR engines better below…
Our out-of-the-box ASR engines are already excellent and ready to use immediately. Our pre-adapted specialized industry domain ASR engines offer even greater accuracy.
For total control and the best accuracy, consider customizing with your own data. Our rapid customization technologies can have your ASR engines adapted to your organizations specific domain in as little as a couple of hours.
Compare the Legacy Approach to Autonomous Speech Recognition
Traditional Legacy Automated Speech Recognition | Autonomous Speech Recognition | |
---|---|---|
Datasets | Large datasets from a small number of speakers | Much larger datasets from a wide variety of speakers |
Hours of Audio | 30,000 | 1,100,000 – 37 x larger |
Labeled Data | Yes | Yes |
Unlabeled Data | No | Yes |
Language Model | Limited | Broad support, notable improvement |
Handling of Accents | Very limited, biased towards Western markets (US, UK, etc.) | Broad support, notable improvement |
Handling of Genders | Limited and biased | Notable improvement |
Handling of Age Variety | Limited and biased | Notable improvement |
Handing of Background Noise | Very limited | Notable improvement |
Understanding Autonomous Speech Recognition
Self-supervised learning has unlocked hundreds of thousands more hours of data to train on. Language Studio ASR learns from a much larger datasets from a huge variety of sources that include both labeled and unlabeled data. Data can be sourced from voice data specialists as well as a broad array of generic data that is readily available on the internet.
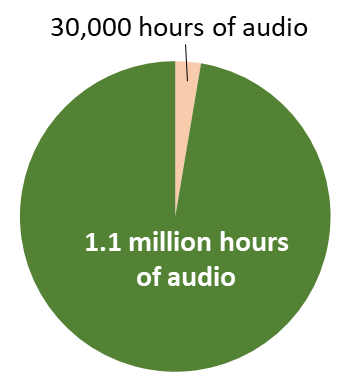
With the addition of language models and the ability to quickly customize your own language model, ASR delivers the highest accruacy of any speech recognition in the market.
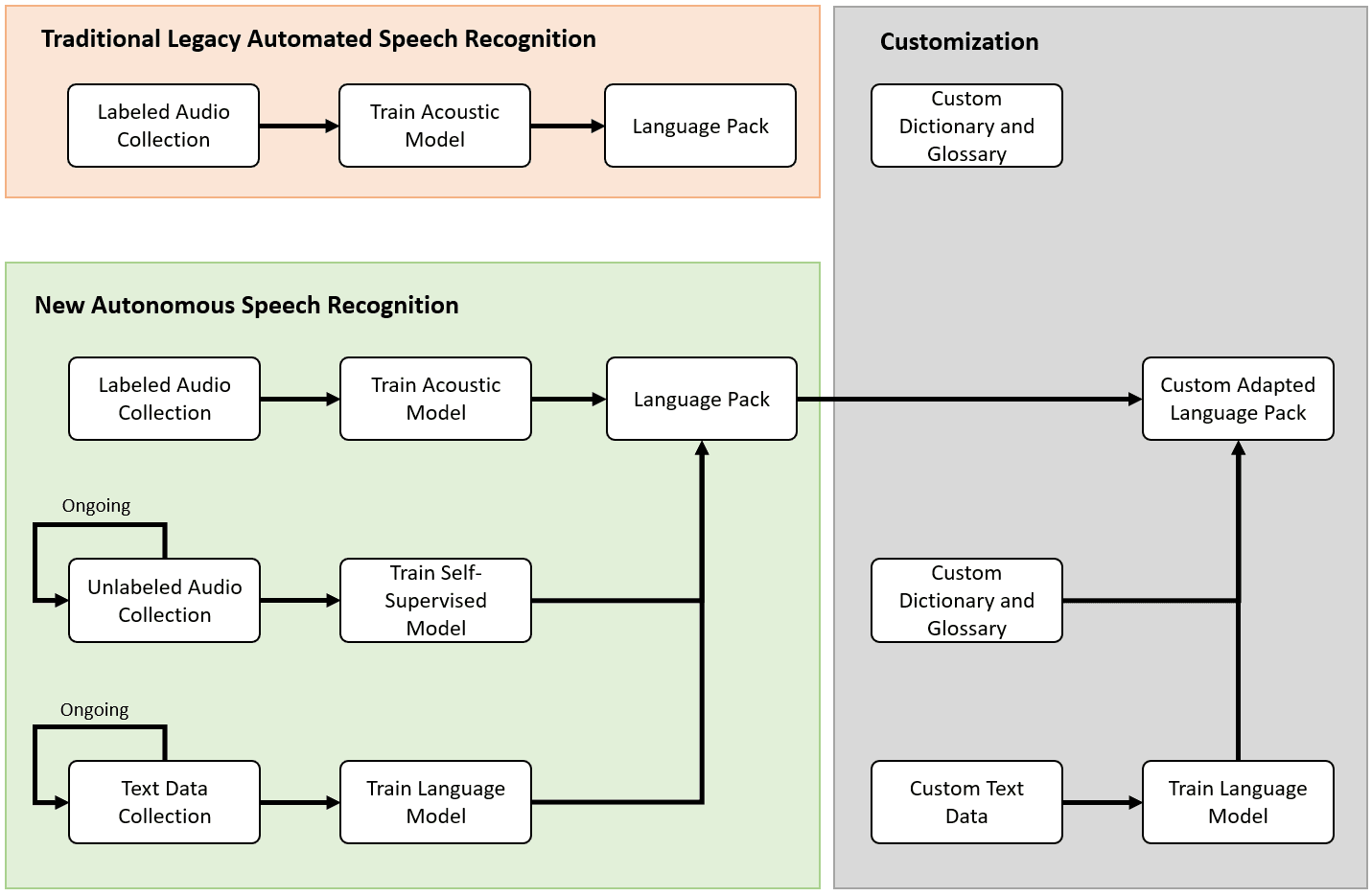
Options for Customizing your ASR Engines
Customizing ASR to your specific use case is a fast process and can be done in just a few days or even a few hours. Customization reinforces context and adds new vocabulary.
Omniscien is preparing a number of off-the-shelf domains that have been pre-customized to specific use cases such as news, finance, medical, etc. This will help to bias the ASR to specific vocabulary and also to teach it quickly new vocabulary.
But sometimes a general domain is not specific enough and may require more specific adaptation. Consider the two news announcements below where the context changes based on the country, but all other words are identical:
England Context:
Prime Minister Boris Johnson went to Wembley today to meet with members of the Weybridge City Council after the recent policy meetings in Cambridge.
Malaysia Context:
Prime Minister Ismail Sabri Yaakob went to Bukit Timah today to meet with members of the Ipoh City Council after the recent policy meetings in Putrajaya.
Both contexts use news with same basic phrase. Each has a different contextual locale. The speech processed in the context of England had very high accuracy due to the data that the ASR was trained with. The speech processed in the context of Malaysia with people names and location names relevant to Malaysia has low accuracy as the Malaysian vocabulary for these names was not included in the initial training data.
Customization is a rapid approach that quickly injects new vocabulary and context to match a specific customer use case such as that above. After customization, the ASR recognized “Ismail Sabri Yaakob”, “Bukit Timah”, “Ipoh”, and “Putrajaya” correctly.
Similarly, for biasing and adding vocabulary in other domains such as Finance or Medical, customization via Domain Adaptation improves the quality of the ASR output considerably.
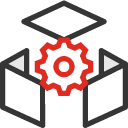
Select a Pre-Trained Industry Specific ASR Engine
We are progressively training pre-built industry domains that match our machine translation industry domains.
Select from Automotive, Banking and Finance, E-Discovery, Engineering and Manufacturing, General Purpose, Information Technology, Medicine and Life Sciences, Military Intelligence and Defense, News and Media, Patents and Legal, Politics and Government, Retail and E-Commerce, Subtitles and Dialog, and Travel and Hospitality.
Each Industry Domain is built on a base of millions of high-quality sentences that are relevant to the context that they represent. Each individual sentence has been processed using a series of analysis and quality validation tools that ensure that only high-quality domain specific text is used to teach the models.
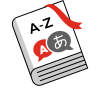
Add Custom Glossary and Dictionary
Specify up to 1,000 custom dictionary/glossary terms per language to fine tune your engine on specific words.
This feature is useful to apply instant changes when you know the vocabulary. This feature is most commonly used for specific product names, brand names, people names or words that the speech recognition is having trouble detecting.
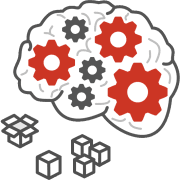
Customized to Your Specific Domain
Omniscien offers a full customization service. We will advise you and guide you on what is the optimal approach to customization your engine.
In some cases you already have sufficient data, we can customize your engine in just a few hours. Simply upload your content and our automated processes will quickly extract the data needed to customizing your ASR engine.
If you don’t have sufficient content, Omniscien has a range to tools for gathering, mining, matching, and collecting content that fits your domain. We will work with you to quickly get the right data to see a notable improvement in accuracy.
Solving Problems while Improving Accuracy and Removing Bias
By leading the industry from Automatic Speech Recognition towards Autonomous Speech Recognition (ASR) and using the latest techniques in machine learning, including the introduction of self-supervised models and advanced language models, we take accuracy to the next level.
This step-change means that Language Studio ASR is able to outperform all of the industry’s legacy players. While ASR is still not perfect, our approach to speech recognition is helping to solve many of the legacy issues and biases that have historically plagued ASR. Our technology is making sure that all voices can be heard.
The numbers speak for themselves (no pun intended). The chart on the right shows just how far ahead in terms of accuracy Language Studio is compared to the industry’s leading players.
With support for 48 languages ranging from Arabic to Welsh, and new languages being added regularly, it is clear that our approach is delivering across every language that we have built. See a list of all the languages currently supported and in development >>
But accuracy comes down to many factors. The sections below demonstrate how our ASR technology is outperforming the rest of the industry in areas such as gender and age bias, accents, and background noise.
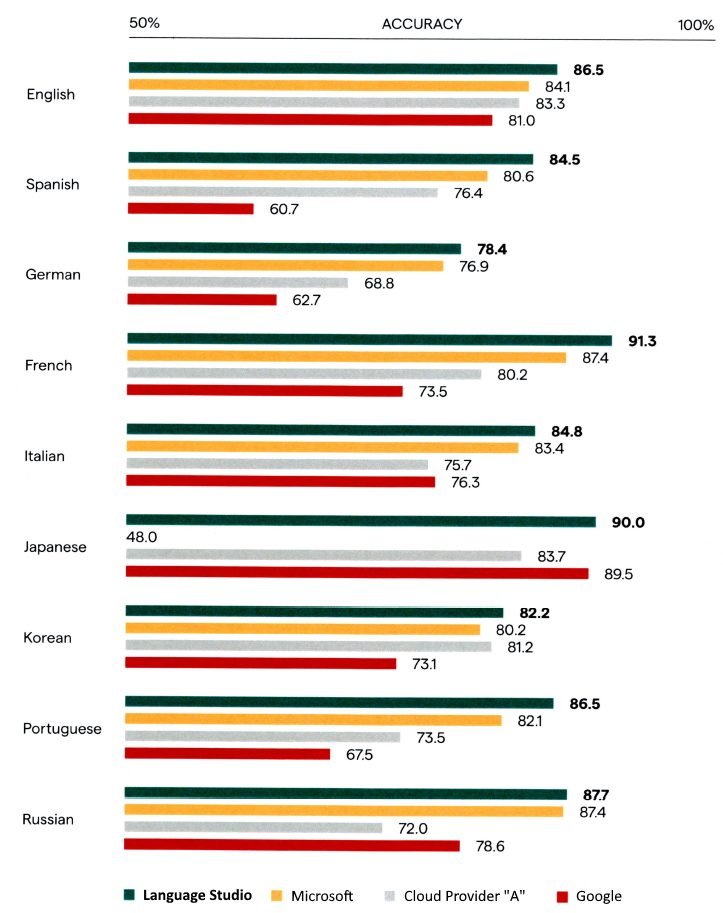
Accents Accuracy
Using the Stanford study as a starting point, we can see just how vast the disparity was with accents. Across the industry, speech recognition performed poorly for African American Vernacular English (AAVE), a couple of major players barely made correct transcriptions for half of the words spoken.
Yet, with the same recordings of Regional African American Language as the Stanford analysis, we found our Autonomous Speech Recognition was far and away the best at improving accuracy amongst those who speak AAVE. While still not perfect, it was magnitudes better than any other speech recognition vendor.
Why was our software so much better? Simply because our ASR technology uses more than a million hours of audio that is not accessible to other vendors due to technology differences. Our self-supervised learning for training approach allows for more data, more accents and more dialects to be covered that ever before.
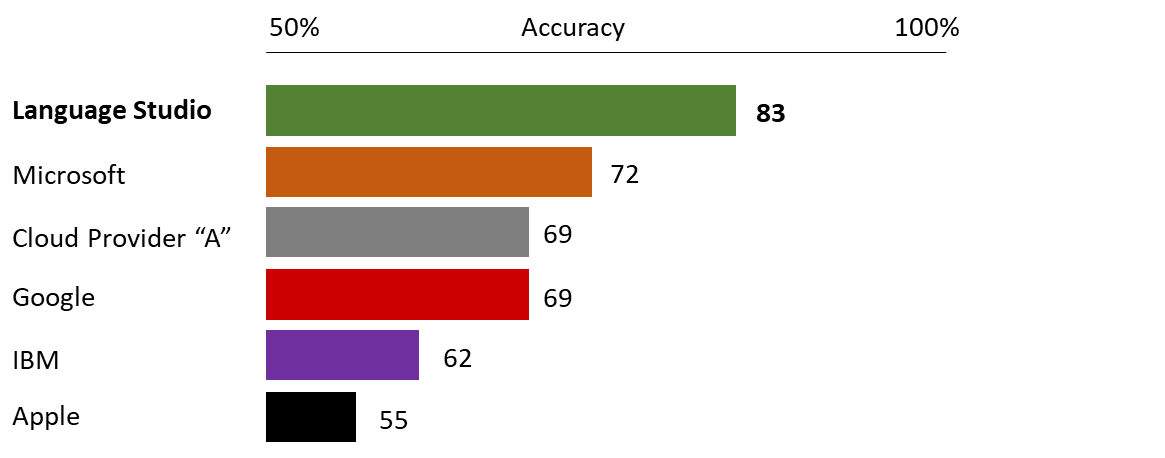
Age Accuracy
In a world of speech recognition – just as with accents – there has been a gap in accuracy between younger and older voices. This was notable accuracy challenges with voices of people under the age of 18, when compared to older voices. Again, the scope of labeled data to learn on has always been an issue, but now working with both labeled and unlabeled data, this bottleneck can be removed.
Our first round of results (using material from the open-source project Common Voice) has shown another fundamental shift in closing this gap.
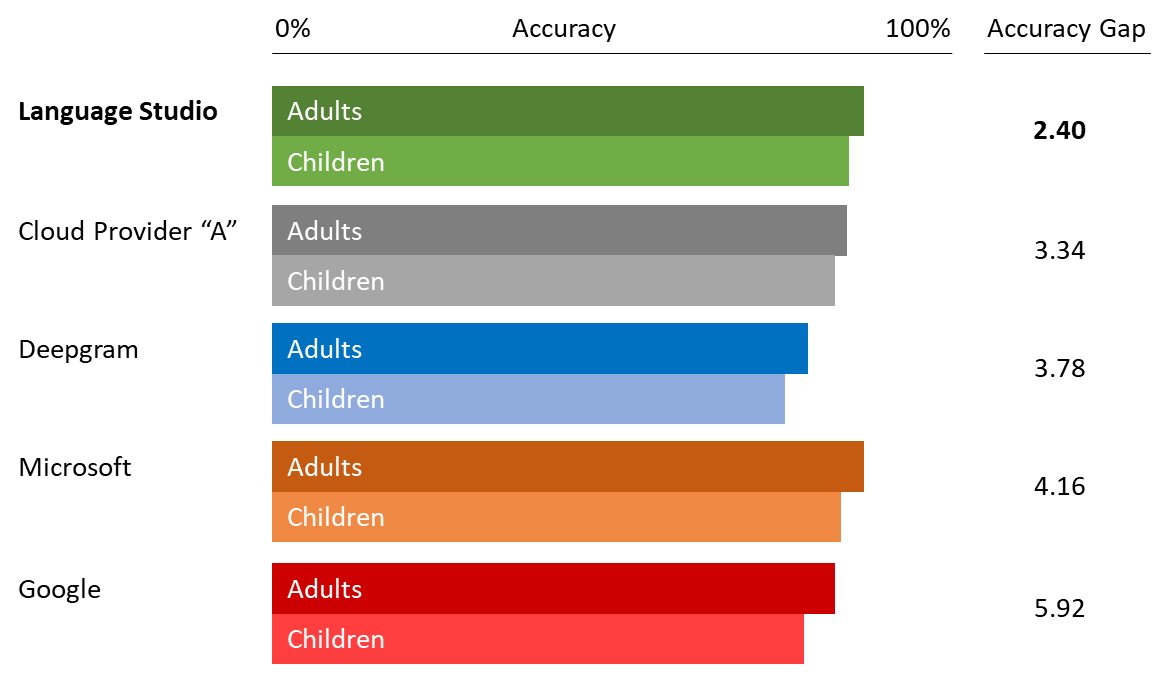
Gender Accuracy
Proving a disparity in the accuracy of speech recognition when it comes to gender has proved difficult. Some tests have shown software sure software delivers an accuracy gap that favors women, some tests show it favors men.With so many other factors to consider – dialect, language, age, etc. – it might take time before we see notable trends. But by training with self-supervised models, we’re hopeful any gap will be minimal whichever way they fall.We will continue to observe gender identity and bias in ASR. As a society and the world at large continues to evolve its thinking, well do everything we can to provide easy to use and accurate speech-to-text software for everyone.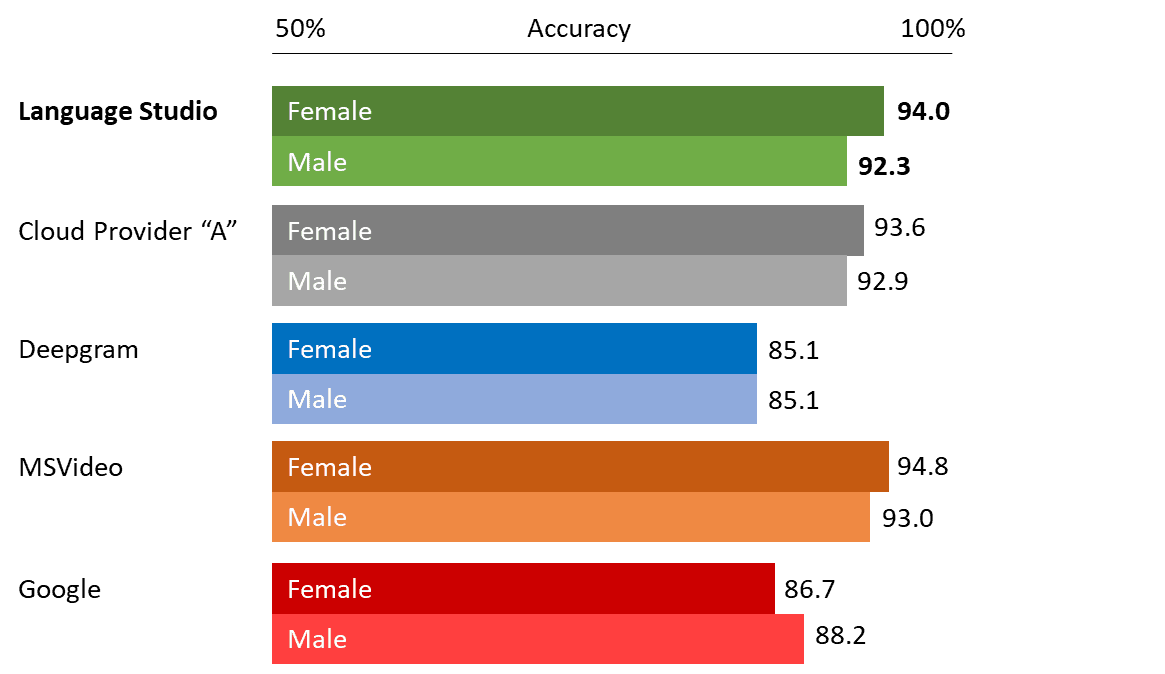
Background Noise Accuracy
An age-old challenge for transcription is the quality of the recordings that we use. Material with background noise has always made it hard to turn speech into text. Even before ASR, poor quality recordings were often the biggest bugbear in human transcription.
Today, organizations are transcribing more content than ever before. Phone calls, webinars, movies, videos, presentations, meetings, and more are transcribed and part of normal business activities. Many have music, loud background noises, or just generally uncontrolled noisy environments.
To truly test our Autonomous Speech Recognition, we wanted to see how it would handle poor quality data. We manipulated 6 hours of audio taken from meetings, earnings calls, online videos, and a host of other real-world examples. When then added ambient sounds (phones, machine noises, other conversations, etc.) and tested our software against some of our competitors. To make it even more of a challenge, we randomly played around with pitch, reverb, and volume levels too.
As with age and accent, the results showed that we were once again above our competition and making yet more promising steps in the right direction.
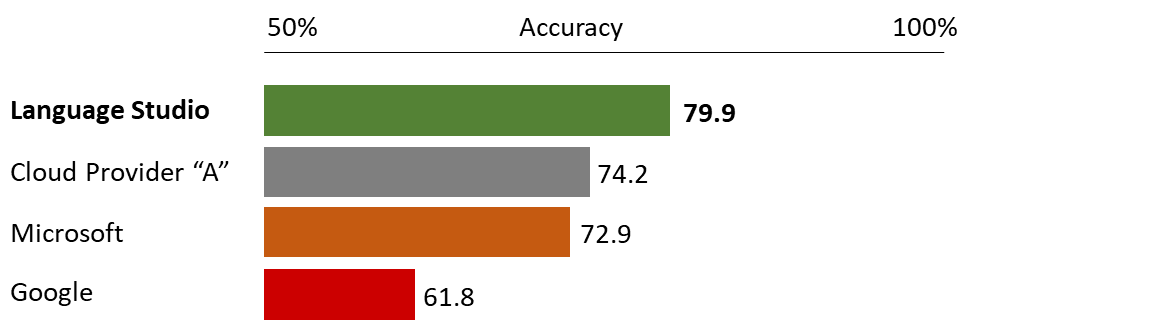